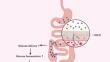
Overview
The Journal of Diabetes & Metabolic Disorders is a peer-reviewed journal publishing original clinical and translational articles and reviews in endocrinology and related areas of research.
- Official Journal of the Endocrinology and Metabolism Research Institute.
- Provides a high-quality forum for debates on relevant issues.
- Editor-in-Chief
-
- Bagher Larijani
- Associate Editor
-
- Fatemeh Bandarian
- Impact factor
- 2.8 (2022)
- 5 year impact factor
- 3.0 (2022)
- Submission to first decision (median)
- 11 days
- Downloads
- 353,759 (2023)
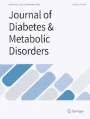
Latest articles
Journal updates
-
World Diabetes Day - Open Access Articles
November 14th marks World Diabetes Day, to mark this day the Journal of Diabetes & Metabolic Disorders would like to showcase a few of their highest cited open access articles on the subject of diabetes
-
Thematic/Special Issue on Precision Medicine
Precision medicine is a newly emerging era and therapeutic strategy that focuses on identifying the most effective therapies based on unique characteristics for the right person at the right time. It is in contrast to a one-size-fits-all approach, in which disease treatment and prevention strategies are developed for general people without consideration of the diversities between individuals.
Journal information
- Electronic ISSN
- 2251-6581
- Abstracted and indexed in
-
- Baidu
- CLOCKSS
- CNKI
- CNPIEC
- Chemical Abstracts Service (CAS)
- Dimensions
- EBSCO
- EMBASE
- Emerging Sources Citation Index
- Gale
- Google Scholar
- IFIS Publishing
- Naver
- OCLC WorldCat Discovery Service
- Portico
- ProQuest
- PubMedCentral
- Reaxys
- SCImago
- SCOPUS
- Semantic Scholar
- TD Net Discovery Service
- UGC-CARE List (India)
- Wanfang
- Copyright information